The NIST has released a new report that suggests that facial recognition providers have gotten much better at identifying people who are wearing masks. The report is a follow-up to a July study that found that many facial recognition algorithms struggled with masked faces.
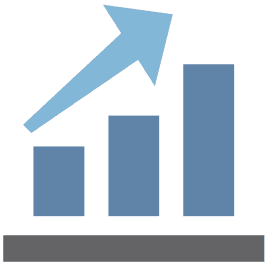
The difference is that the second study includes algorithms that were developed after the onset of COVID-19. The first study, on the other hand, looked only at algorithms that were already available before the pandemic, and that had not yet been optimized to account for covered faces.
The new report has been titled Ongoing Face Recognition Vendor Test (FRVT) Part 6B: Face Recognition Accuracy with Face Masks Using Post-COVID-19 Algorithms. It includes 65 algorithms that were not a part of the first study, bringing the total number to 152. The algorithms were submitted voluntarily, though the NIST did not ask whether or not they were designed for masked faces, or whether they were being featured in commercial products.
The second study used the same methodology and the same 6.2 million-image database that it used the first time around. In that regard, the NIST only conducted one-to-one testing, while the masks themselves were applied digitally in a variety of shapes and colors.
The tests ultimately showed that a handful of the new algorithms performed well with masked and unmasked faces, and could automatically detect differences between the two.
“Some newer algorithms performed significantly better than their predecessors,” said the NIST’s Mei Ngan, one of the authors of the study. “In some cases, error rates decreased by as much as a factor of 10 between their pre- and post-COVID algorithms. In the best cases, software algorithms are making errors between 2.4 and 5% of the time on masked faces, comparable to where the technology was in 2017 on nonmasked photos.”
Despite those improvements, there were still notable issues with some of the other algorithms in the sample. For example, the NIST found that false positives went up dramatically when both the stored image and the test image featured a masked face, which means that organizations should still be using an uncovered face as their saved template for each individual. Meanwhile, the algorithms had more trouble with red and black masks than they did with white and blue ones, and there were fewer errors with smaller masks than there were with large ones. Those trends are in keeping with the organization’s findings from July.
Many facial recognition providers have updated their algorithms during the pandemic, and now claim to be able to identify masked faces just as accurately as their unmasked counterparts. The NIST indicated that it will evaluate the algorithms’ one-to-many capabilities in future rounds of mask testing.
–
December 8, 2020 – by Eric Weiss
Follow Us