A new paper, titled “Generating Automatically Print/Scan Textures for Morphing Attack Detection Applications,” explores innovative methods to enhance Morphing Attack Detection (MAD) systems, offering important applications in biometrics and identity security. The authors, Juan E. Tapia, Maximilian Russo, and Christoph Busch, are affiliated with the Hochschule Darmstadt, specifically with the da/sec-Biometrics and Internet Security Research Group, which is based in Darmstadt, Germany.
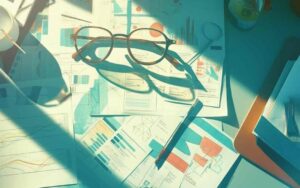
Morphing attacks, which involve blending the facial features of two individuals to create a single, convincing image, pose a significant threat to security, especially in scenarios where physical photographs are submitted for official documents. The paper focuses on overcoming the limitations of existing MAD systems, primarily the scarcity of large and diverse datasets that include printed and scanned images.
To address this issue, the authors propose two novel methods for generating synthetic print/scan images, thereby expanding the training datasets available for MAD systems. The first method leverages advanced image-to-image transfer techniques, specifically the Pix2pix and CycleGAN algorithms. These algorithms enable the creation of synthetic images that closely mimic the variations and artefacts introduced during the printing and scanning processes. By using these techniques, the authors were able to generate a wide range of realistic images, significantly increasing the size and diversity of the datasets used to train MAD algorithms.
The second method is a semi-automatic texture-transfer approach, which isolates the specific textures and artefacts that result from the print/scan process and applies them to digital images. This method allows for the creation of a diverse set of synthetic images that replicate the imperfections commonly found in printed and scanned documents. This approach is particularly valuable because it can be easily applied across different devices and paper types, enhancing the robustness of the generated datasets.
The effectiveness of these methods is demonstrated through extensive evaluations, in which the authors compare the performance of MAD systems trained on both traditional and synthetic datasets. The results are compelling: when using the proposed synthetic data, the MAD system achieved an Equal Error Rate (EER) as low as 1.92 percent on the FRGC/FERET database when trained with the CycleGAN-generated images, compared to higher error rates when using only traditional data. In contrast, the traditional approach without synthetic augmentation often resulted in significantly higher EERs, underlining the effectiveness of the proposed methods.
The paper also highlights the Frechet Inception Distance (FID) as a key metric for evaluating the similarity between synthetic and real images. The best-performing model, based on CycleGAN with ResNet-50 architecture, achieved an FID score of 6.11 for bona fide images, indicating a high degree of realism in the generated images. The semi-automatic texture-transfer method also showed strong results, with an FID of 35.94 for bona fide images, further supporting its utility in generating realistic training data.
The research presents a significant advancement in the field of biometric security by offering scalable and efficient methods for generating large, realistic datasets for MAD systems. These methods not only improve detection accuracy, with EERs as low as 1.92 percent, but also demonstrate the potential for synthetic data to overcome the limitations of traditional dataset generation, thereby enhancing the security of biometric systems against morphing attacks.
The paper is available in pre-print through arXiv.
Source: arXiv
–
August 30, 2024 – by Cass Kennedy
Follow Us